Unsupervised learning#
Learning objectives#
Recap what unsupervised learning is
Explain how this relates to supervised learning, especially the main differences
Perform K-means clustering, a simple unsupervised learning algorithm, on sample data
Recap#
In unsupervised learning, models learn from unlabelled data (i.e. without explicit supervision).
The goal is to learn (often hidden) patterns or structures in the data.
We use unsupervised learning for clustering, dimensionality reduction, anomaly detection, and more.
K-means clustering#
This type of algorithm tries to assign class labels (i.e. generate clusters) from unlabelled data.
There are many different clustering algorithms, but K-means is a great place to start.
How does it work?#
The algorithm is quite simple. To generate k clusters from a dataset, it minimises the variance within each cluster.
Create k cluster centroids randomly
Assign each data point to the nearest centroid
Compute new centroids as the mean of the assigned points
Repeat steps 2 and 3 until the centroids stabilise (i.e. they do not move significantly)
omput
import numpy as np
import matplotlib.pyplot as plt
from sklearn.cluster import KMeans
from sklearn.datasets import make_blobs
# Generate some data
X, y = make_blobs(n_samples=300, centers=4, cluster_std=1.0, random_state=42)
plt.scatter(X[:, 0], X[:, 1], s=10)
plt.title("Raw data")
plt.xlabel('Feature 1')
plt.ylabel('Feature 2')
plt.show()
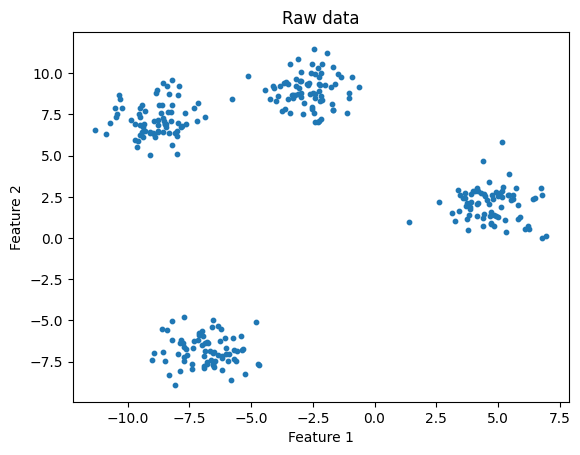
def kmeans_and_plot(X, n_clusters, title):
# Apply k-means
kmeans = KMeans(n_clusters, random_state=42)
kmeans.fit(X)
y_kmeans = kmeans.predict(X)
# Plot data coloured by k-means
plt.scatter(X[:, 0], X[:, 1], c=y_kmeans, s=10, cmap="viridis")
plt.scatter(
kmeans.cluster_centers_[:, 0],
kmeans.cluster_centers_[:, 1],
c="red",
marker="X",
s=100,
label="Centroids",
)
plt.title(title)
plt.xlabel('Feature 1')
plt.ylabel('Feature 2')
plt.legend()
plt.show()
n_clusters = 4
title="K-means: Raw data coloured by predicted cluster"
kmeans_and_plot(X, n_clusters, title)
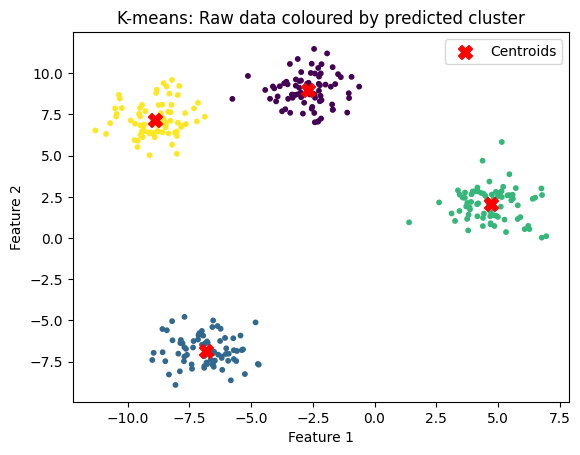
What happens if we have the wrong k?#
Here we could easily see 4 clusters, and specify k = 4.
But what if we provide the wrong k?
n_clusters = 2
title="K-means: wrong number of clusters (too few)"
kmeans_and_plot(X, n_clusters, title)
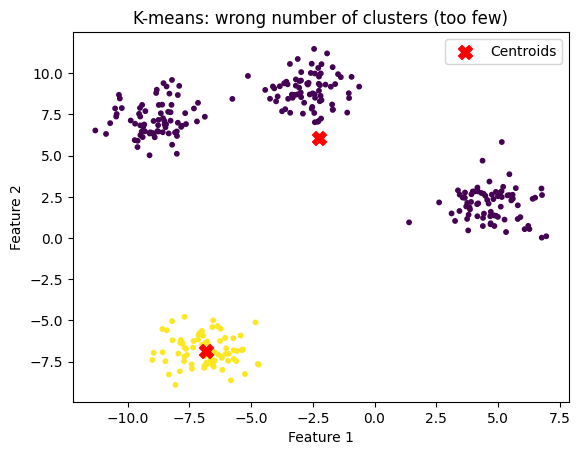
n_clusters = 10
title="K-means: wrong number of clusters (too many)"
kmeans_and_plot(X, n_clusters, title)
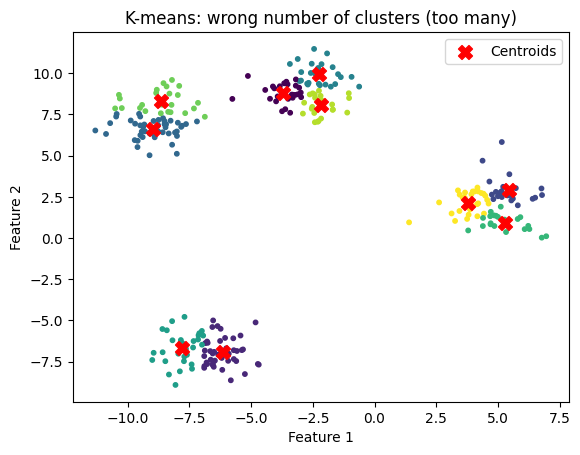
Finally, what happens if we have a less distinct data set?
# Generate some data
X, y = make_blobs(n_samples=500, centers=10, cluster_std=2.0, random_state=42)
plt.scatter(X[:, 0], X[:, 1], s=10)
plt.title("Raw data")
plt.xlabel('Feature 1')
plt.ylabel('Feature 2')
plt.show()
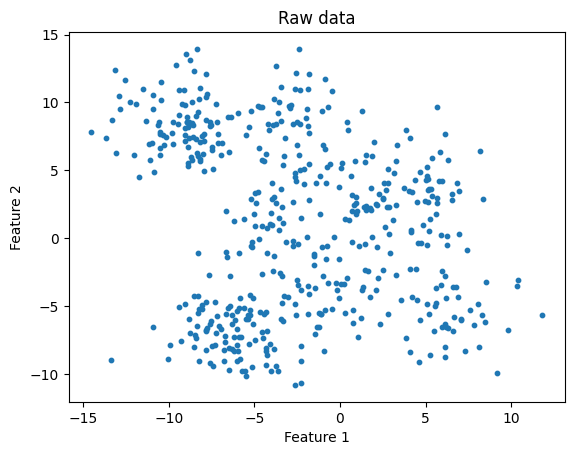
n_clusters = 10
title="K-means: correct number of clusters, less distinct clusters"
kmeans_and_plot(X, n_clusters, title)
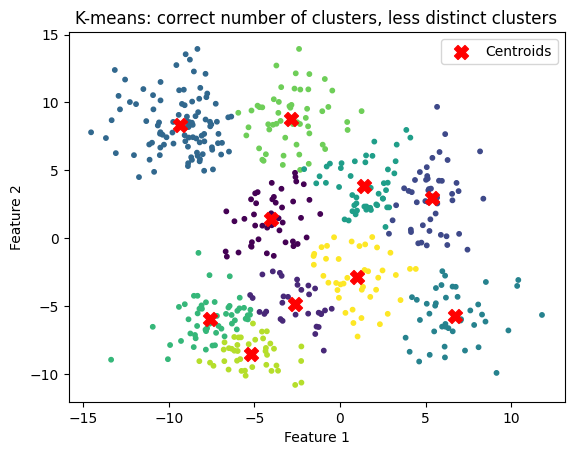
Determining the number of clusters#
We can attempt to calculate the number of clusters using the elbow method.
This is not perfect, as shown below
# Generate some data
X, y = make_blobs(n_samples=500, centers=6, cluster_std=1., random_state=32)
plt.scatter(X[:, 0], X[:, 1], s=10)
plt.title("Raw data")
plt.xlabel('Feature 1')
plt.ylabel('Feature 2')
plt.show()
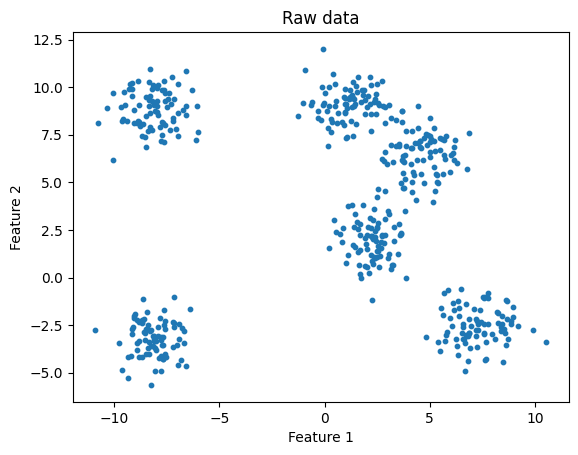
def elbow_method_and_plot(X, k_range, title, random_state=42):
# Store within cluster sum of squares (inertia)
wcss = []
for k in k_range:
kmeans = KMeans(n_clusters=k, random_state=random_state)
kmeans.fit(X)
# Store inertia
wcss.append(kmeans.inertia_)
# Plot the elbow curve
plt.figure(figsize=(8, 5))
plt.plot(k_range, wcss, marker='o', linestyle='-')
plt.xlabel("Number of clusters k")
plt.ylabel("Within cluster sum of squares (WCSS)")
plt.title(title)
plt.xticks(k_range)
plt.show()
k_range = range(1, 11)
title = "Elbow method for finding k"
elbow_method_and_plot(X, k_range, title, random_state=42)
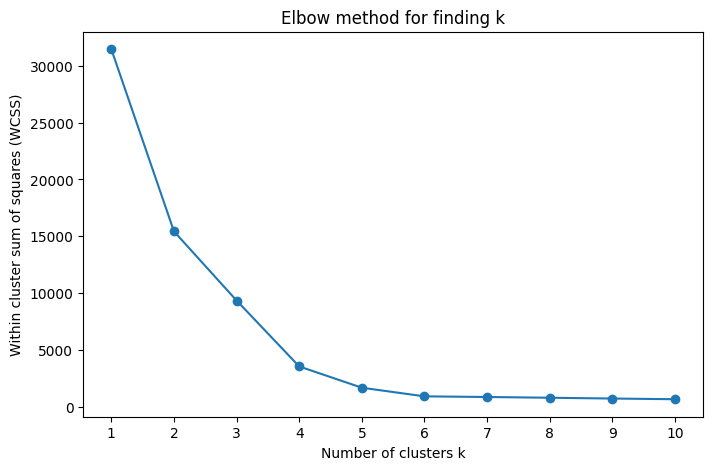
n_clusters = 5
title="K-means: data colorued by elbow method"
kmeans_and_plot(X, n_clusters, title)
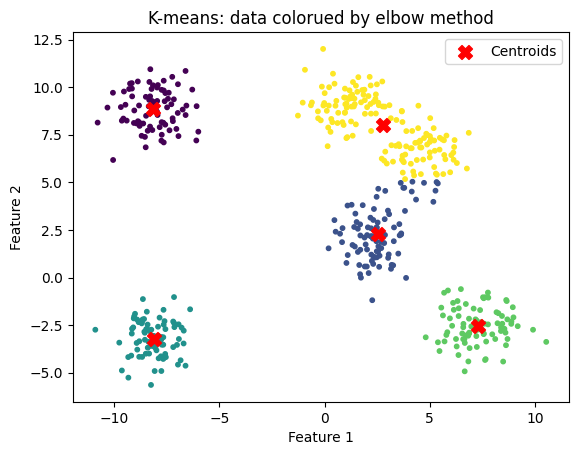
When the clusters are less distinct, the elbow method might be less helpful:
# Generate some data
X, y = make_blobs(n_samples=500, centers=6, cluster_std=2, random_state=32)
plt.scatter(X[:, 0], X[:, 1], s=10)
plt.title("Raw data: 6 clusters (apparently)")
plt.xlabel('Feature 1')
plt.ylabel('Feature 2')
plt.show()
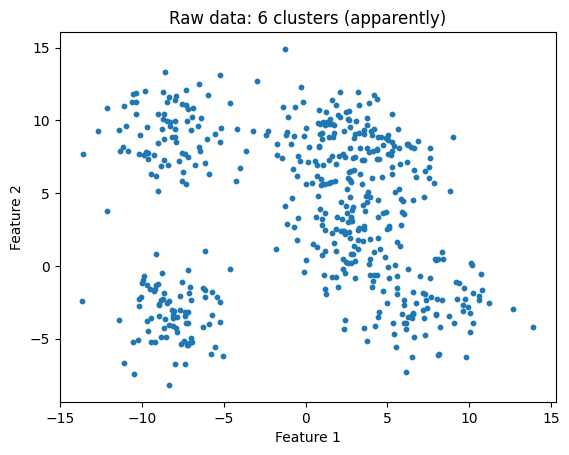
k_range = range(1, 11)
title = "Elbow method for finding k"
elbow_method_and_plot(X, k_range, title, random_state=42)
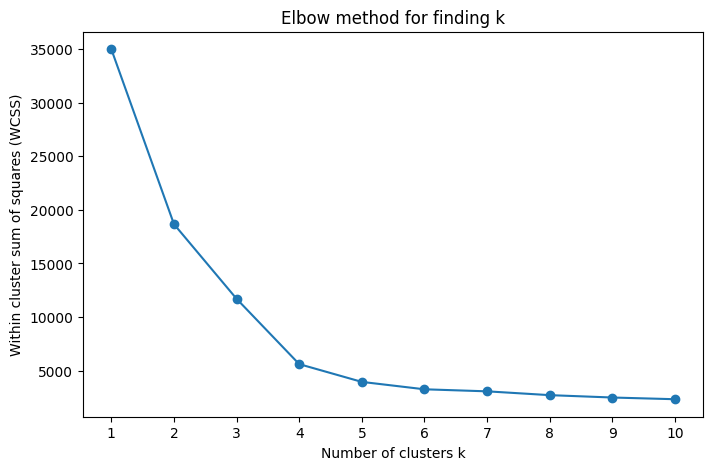
n_clusters = 4
title = "K-means: data coloured by elbow method"
kmeans_and_plot(X, n_clusters, title)
